Understanding the Importance of Bounding Boxes in Data Annotation
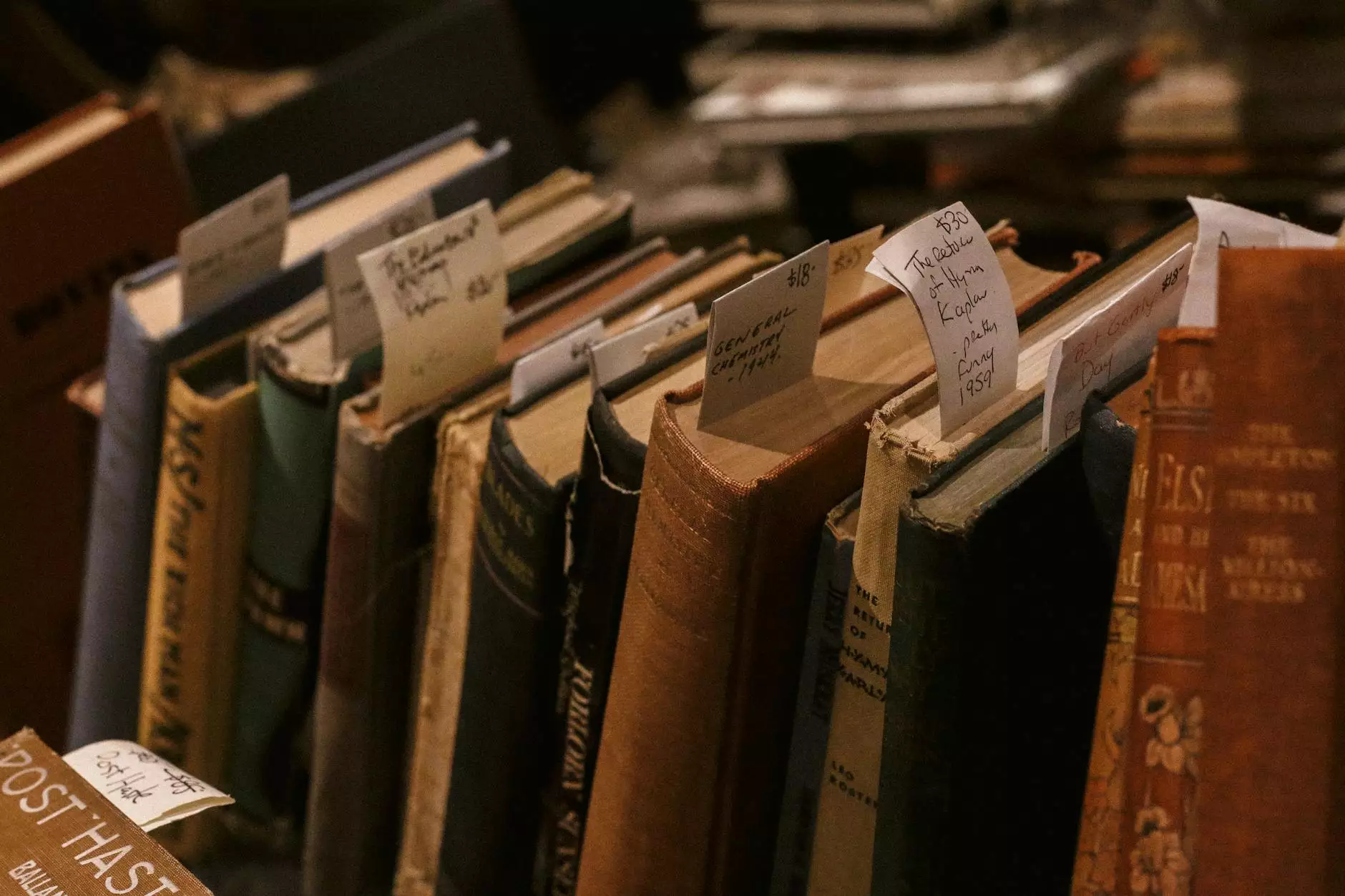
In the ever-evolving landscape of artificial intelligence (AI) and machine learning, data annotation plays a crucial role in developing intelligent systems capable of understanding and interpreting the world around them. At the forefront of this process is the concept of bounding boxes, a vital component in the annotation toolkit. This article delves into the significance of bounding boxes, their applications, and how businesses like Keylabs.ai utilize them to enhance their data annotation services.
What Are Bounding Boxes?
Bounding boxes are rectangular graphs used to specify the location of an object within an image or a video frame. They serve as a straightforward yet effective method for image annotation, allowing AI models to perceive the spatial dimensions and position of various objects. By outlining the perimeter of an object, these bounding boxes provide vital information for supervised learning, enabling algorithms to recognize and differentiate between numerous objects in a visual context.
The Role of Bounding Boxes in Data Annotation
The data annotation process includes various techniques, but the use of bounding boxes is particularly prevalent due to their effectiveness and accuracy. Here’s a look at the key roles they fulfill:
- Object Detection: Bounding boxes are pivotal for training machine learning models in object detection tasks. They help the models learn to identify and classify objects by showing where an object is located within an image.
- Image Segmentation: Beyond simple object recognition, bounding boxes assist in image segmentation, where the goal is to classify each pixel in the image. Bounding boxes mark regions of interest that can be processed further.
- Efficient Data Annotation: By providing a concise way to denote object boundaries, bounding boxes help reduce the time and resources required for data annotation, making it quicker to compile datasets.
Why Bounding Boxes Matter in AI Development
In the context of AI and machine learning, the efficacy of the models largely depends on the quality of the training data. Here are some reasons why bounding boxes are integral to effective AI development:
1. Enhanced Precision in Object Recognition
When ML models are trained with well-annotated images, they become better at recognizing objects with high precision. Bounding boxes provide a clear demarcation of objects, thus enabling models to focus on the most relevant features of an object during training.
2. Facilitating Complex Tasks
In addition to basic object recognition, bounding boxes allow machines to perform more complex tasks such as tracking moving objects in video feeds, which is essential in applications like autonomous driving, security surveillance, and many more. The clear delineation of objects is what facilitates these intricate functionalities.
3. Enabling Transfer Learning
Bounding boxes allow for the use of transfer learning, a process where a model trained on one task is adapted for another task. For instance, a model trained to recognize cars in bounding boxes can be fine-tuned to recognize different types of vehicles with minimal additional annotation.
Implementing Bounding Boxes in Data Annotation Projects
Companies looking to implement data annotation projects need to ensure they use bounding boxes effectively. Here are key guidelines for implementing bounding boxes:
- Consistency: When annotating objects with bounding boxes, consistency in the box size and placement is critical. This uniformity aids the machine learning model in learning effectively from the training data.
- Quality Control: Regular quality checks should be instituted to ensure that the bounding boxes align accurately with the objects they represent. Inaccurate annotations can lead to poor model performance.
- Tool Selection: Choosing the right data annotation tools is vital. Platforms like Keylabs.ai offer advanced tools for bounding box annotation with features that support high-speed and high-quality output.
The Impact of Bounding Box Annotation on Industries
Bounding box annotation has profound implications across various industries. Here's how specific sectors benefit:
1. Automotive
In the automotive sector, especially with the rise of autonomous vehicles, accurate bounding box annotation is crucial for the recognition of pedestrians, road signs, vehicles, and other important elements that contribute to safe driving.
2. Healthcare
Bounding boxes help in medical imaging, where specific areas of interest—like tumors or other anomalies—can be accurately outlined. This aids in diagnostics and enhances the efficacy of AI systems in healthcare.
3. Retail
In retail, bounding box annotations facilitate inventory management systems, enabling automated systems to detect products on shelves, assess stock levels, and enhance customer experience through AI-driven solutions.
Keylabs.ai: Leading the Way in Data Annotation
Keylabs.ai stands out in the realm of data annotation services, providing exceptional solutions powered by advanced technologies. Here’s what makes their approach to using bounding boxes unique and beneficial:
- Scalability: The platform is designed to handle projects of varying sizes efficiently, making it suitable for startups and large enterprises alike.
- Advanced Annotation Tools: Keylabs.ai offers sophisticated tools that streamline the bounding box annotation process, ensuring faster and more accurate results.
- Expert Team: A skilled workforce ensures that the annotations maintain high standards, which is crucial for training robust AI models.
Conclusion: The Future of Bounding Boxes in Data Annotation
Bounding boxes will continue to be an essential part of data annotation as AI and machine learning technologies advance. Companies leveraging Keylabs.ai's state-of-the-art data annotation tools will thus find themselves at the forefront of AI innovation. As the demand for accurate and efficient data annotation solutions grows, understanding the importance of bounding boxes will be crucial for any business aiming to succeed in the technology-driven landscape.
Ultimately, adopting robust data practices, including effective use of bounding boxes, will empower industries to harness the full potential of AI, driving growth and innovation across diverse fields.