Harnessing Healthcare Datasets for Machine Learning
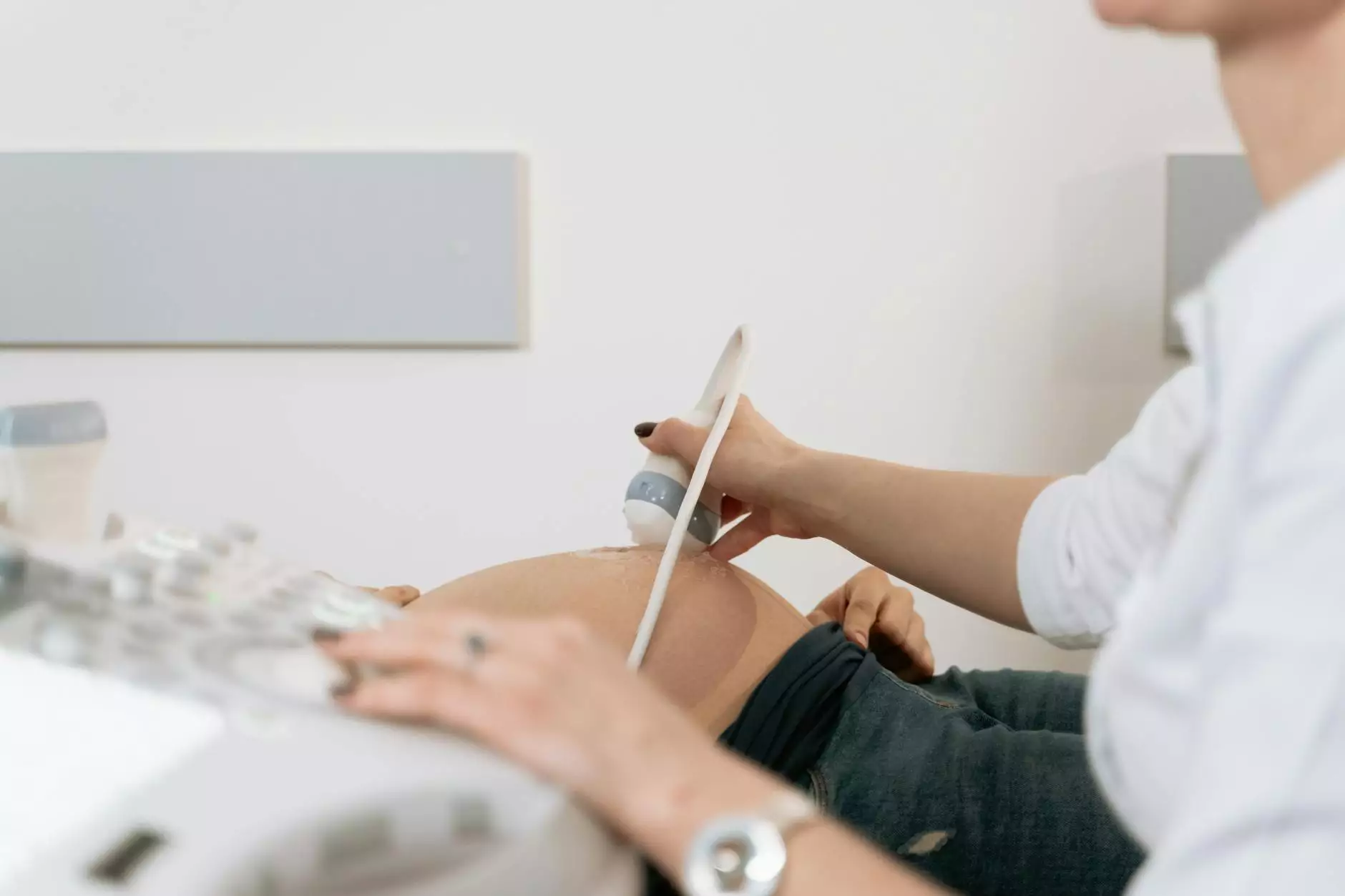
Understanding the Intersection of Healthcare and Technology
In an era where data is king, the role of healthcare datasets for machine learning stands out as a beacon of innovation. These datasets are transforming the landscape of healthcare delivery, driving research, enhancing patient outcomes, and improving efficiencies across systems. The healthcare sector, notorious for its complexity and data richness, is now reaping the benefits of machine learning technologies that leverage vast amounts of data to derive meaningful insights.
The Importance of Healthcare Datasets in Machine Learning
Machine learning relies heavily on data to identify patterns and make decisions. Here, healthcare datasets serve crucial roles:
- Predictive Analytics: By analyzing historical patient data, machine learning models can forecast future health issues, allowing for preventative care.
- Personalized Medicine: Machine learning algorithms can examine genetic information alongside treatment history to tailor personalized treatment plans.
- Operational Efficiency: Datasets can streamline hospital operations, optimizing scheduling, resource allocation, and patient flow.
- Clinical Decision Support: With advanced analytics, healthcare practitioners can receive real-time recommendations, improving diagnosis accuracy.
- Drug Discovery: Machine learning can analyze vast chemical datasets rapidly, accelerating the drug discovery process.
Types of Healthcare Datasets Utilized in Machine Learning
Various types of healthcare datasets for machine learning are pivotal in driving innovation within the sector. One can categorize these datasets into several types:
1. Electronic Health Records (EHR)
EHRs are digital versions of patients’ paper charts and are a major source of data for machine learning. They contain comprehensive histories regarding patients’ diagnoses, treatment plans, medications, immunization dates, allergies, radiology images, and laboratory test results.
2. Clinical Trial Data
Data collected from clinical trials provides robust datasets for analysis. This information helps assess the effectiveness and safety of medical treatments, drawing insights from diverse populations.
3. Patient Surveys and Questionnaires
Incorporating subjective responses from patients via surveys, this data plays a vital role in understanding patient satisfaction and outcomes, thereby enhancing the quality of care.
4. Genomic Data
With advancements in genetic sequencing technologies, genomic data represents a growing dataset that can pave the way for breakthroughs in personalized medicine.
5. Wearable Device Data
Wearable technology has revolutionized health monitoring. Data collected from devices such as fitness trackers and smartwatches provide real-time insights into patients’ daily activities, heart rate, and other vital signs.
Challenges in Harnessing Healthcare Datasets
Despite the enormous potential, utilizing healthcare datasets for machine learning comes with challenges:
- Data Privacy and Security: Patient confidentiality must be maintained, adhering to regulations such as HIPAA in the US, making data sharing complex.
- Data Quality: Inconsistent data collection methods can lead to inaccuracies, affecting the reliability of the machine learning models.
- Integration of Diverse Data Sources: Seamless integration of varied types of healthcare datasets can pose technical challenges.
- Bias in Data: If certain populations are underrepresented in datasets, AI systems may produce biased outcomes, perpetuating health disparities.
Case Studies: Success Stories of Machine Learning in Healthcare
Real-world applications of machine learning utilizing healthcare datasets have yielded remarkable results:
1. Predicting Patient Readmission
Healthcare providers have successfully used machine learning algorithms to predict which patients are at risk of readmission within 30 days post-discharge. By analyzing a variety of factors including demographics, medical history, and treatment details, hospitals can identify high-risk patients and tailor follow-up care accordingly, thereby reducing readmission rates and saving costs.
2. Early Detection of Diseases
Machine learning has been instrumental in the early detection of diseases such as diabetes and cancer. For instance, algorithms that analyze patient records and imaging data have shown improved accuracy and speed in identifying tumors, leading to earlier interventions and better prognosis.
3. Reducing Drug Development Time
Pharmaceutical companies are harnessing machine learning to analyze vast data sets of clinical data, aiming to discover which compounds are most likely to succeed. This approach dramatically cuts down research timelines, leading to faster, cost-effective drug development.
Future Directions of Machine Learning in Healthcare
The future of healthcare is inextricably linked to the advancement of machine learning technologies. Potential directions include:
1. Enhanced Predictive Analytics
With continuous improvements in algorithms and data collection methods, the predictive abilities of machine learning models will enhance, leading to proactive rather than reactive care approaches.
2. Improved Interoperability
Efforts to achieve seamless interoperability among diverse health information systems will facilitate broader data sharing, enhancing machine learning applications.
3. Expansion of Telehealth
As telemedicine gains traction, the integration of machine learning can improve diagnostic accuracy and patient monitoring in virtual healthcare settings.
4. Personalized Health Experiences
Machine learning is expected to play a pivotal role in developing customized healthcare experiences, allowing for treatments tailored not only to individual genetics but also lifestyle, preferences, and socio-economic factors.
Conclusion
As we stand on the brink of a new era in healthcare, the application of healthcare datasets for machine learning holds the promise of a healthier future for individuals and societies alike. By overcoming existing challenges and harnessing the potential of these technologies, healthcare providers can cultivate a system that not only treats illnesses but actively enhances the quality of life through predictive and personalized care.
© 2023 Keymakr - Home Services, Keys & Locksmiths