Understanding Bounding Boxes in Data Annotation: Boost Your Business with KeyLabs
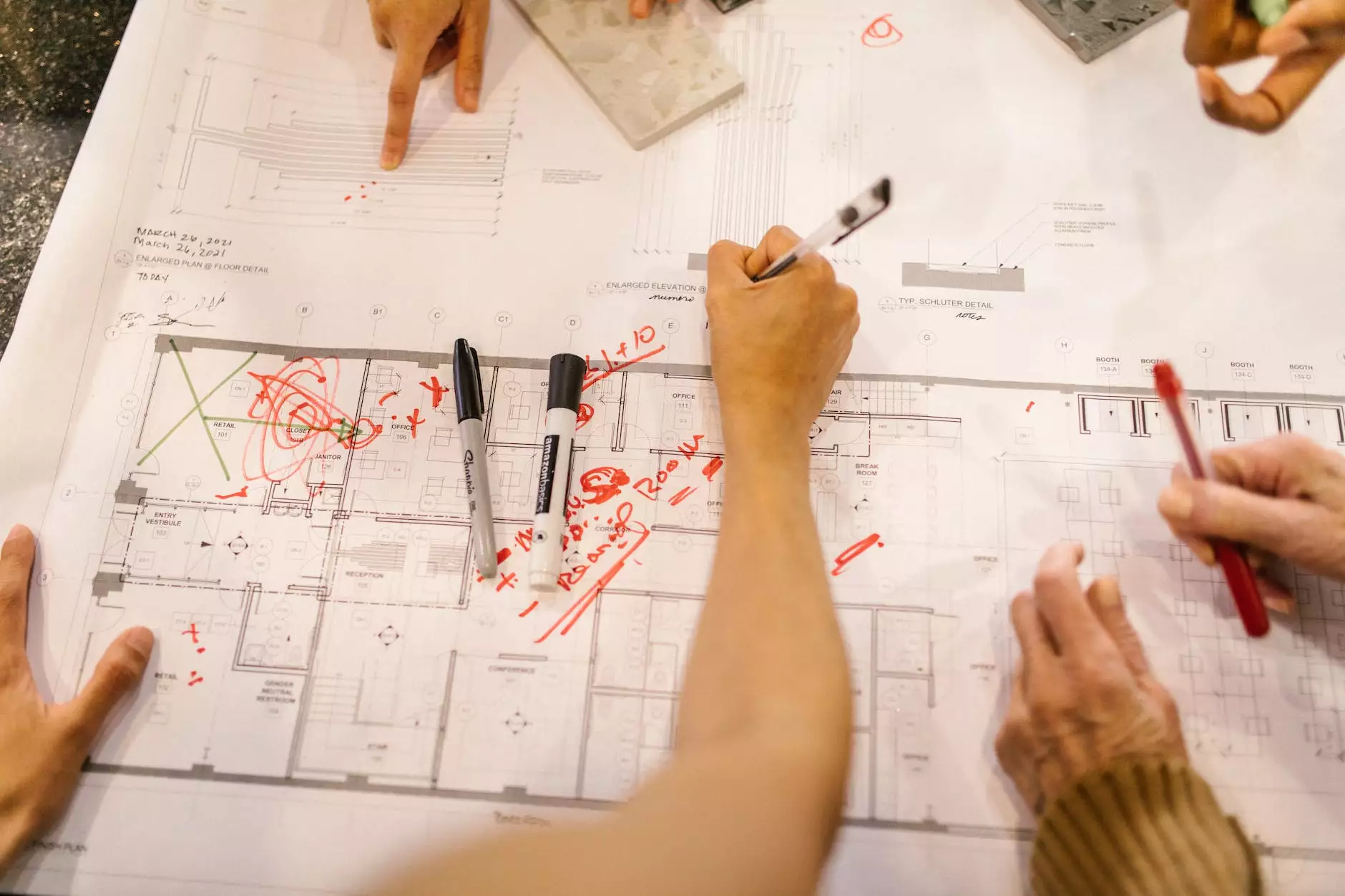
In the world of data annotation, the term "bounding boxes" plays a crucial role in effectively training machine learning models. As businesses increasingly seek to utilize artificial intelligence for enhanced decision-making and operational efficiency, understanding how bounding boxes function within data annotation platforms is essential. This article delves into the significance of bounding boxes, their applications, and how businesses can leverage them through KeyLabs.
What Are Bounding Boxes?
A bounding box is a rectangular box that is drawn around an object in an image or video. This box defines the extent of the object within the frame, providing a precise area that machine learning algorithms can recognize and analyze. Bounding boxes are an essential part of the data annotation process, particularly in computer vision, as they serve multiple purposes including:
- Object Detection: Identifying where specific objects are located within a larger set of data.
- Image Segmentation: Helping algorithms distinguish different objects or parts of an image.
- Training AI Models: Providing labeled data that machine learning models require to learn and improve accuracy.
The Importance of Bounding Boxes in Data Annotation
Bounding boxes are vital for effective data annotation processes. They offer a straightforward way to convey information about objects within an image, which can significantly improve a model's performance. Here are some reasons why bounding boxes are important:
1. Enhanced Object Recognition
Accurate segmentation and identification of objects lead to better recognition rates. When data is annotated with bounding boxes, it allows machine learning algorithms to learn how to identify and classify objects based on clear visual cues.
2. Scalability
When dealing with large datasets, bounding boxes enable scalable annotation methods. Rather than manually outlining every pixel of an object, annotators can simply draw bounding boxes, thereby saving time and resources.
3. Versatility Across Applications
The application of bounding boxes extends across various sectors. From autonomous vehicles to medical imaging, the use of bounding boxes is extensive, making them a flexible tool for data annotation across different domains:
- Autonomous driving systems require bounding boxes to detect pedestrians, vehicles, traffic signs, and more.
- In the healthcare sector, bounding boxes help identify tumors or abnormalities in medical scans.
- Retail businesses use bounding boxes to analyze customer interactions with products in images or videos for targeted marketing strategies.
How to Create Effective Bounding Boxes
While bounding boxes serve a clear function, the effectiveness of their implementation matters significantly. Here are some best practices for creating effective bounding boxes:
1. Precision is Key
Ensuring that the bounding box tightly fits the object without excess space is crucial. Too loose or too tight bounding boxes can mislead the AI, leading to poor performance.
2. Consistency in Annotation
Maintaining a consistent approach to how bounding boxes are drawn can help create a robust dataset. Setting guidelines for annotators can ensure uniformity, which is critical for model training.
3. Quality Control Measures
Implementing thorough quality checks on annotated data can prevent errors from being propagated during model training. Using statistical methods to assess the accuracy of bounding boxes can be beneficial.
Bounding Boxes in Action: Real-World Examples
To further grasp the significance of bounding boxes, let us explore some real-world applications where bounding boxes play a pivotal role:
1. Facial Recognition Systems
Bounding boxes are commonly utilized in facial recognition technology. They help delineate a person’s face in images, allowing algorithms to analyze the facial features distinctively. By training models on a dataset with well-annotated bounding boxes, businesses can enhance security systems and personalize user experiences.
2. Surveillance Systems
In security and surveillance, bounding boxes can indicate suspicious activity by capturing and highlighting subjects of interest in a video feed. Automated systems using bounding boxes can alert security personnel based on predefined parameters.
3. E-commerce Image Tagging
E-commerce platforms leverage bounding boxes to categorize and tag product images. It ensures that products are easily searchable as they are clearly identified through visual data, enhancing the customer experience.
Choosing the Right Data Annotation Tool
Selecting the right data annotation tool is fundamental to successfully integrating bounding boxes into your business processes. KeyLabs offers exceptional features that cater to the needs of businesses looking to annotate data efficiently:
- Intuitive Interface: KeyLabs provides a user-friendly interface for annotators, simplifying the process of drawing bounding boxes.
- Collaboration Tools: Easy collaboration features enhance teamwork among data annotators, ensuring consistency and accuracy.
- Quality Assurance: KeyLabs incorporates built-in quality assurance checks to validate the accuracy of bounding boxes.
- Scalable Solutions: Whether your needs are big or small, KeyLabs can accommodate all with scalable solutions tailored to your business.
Integrating Bounding Boxes Into Your Business Model
Embracing data annotation and bounding boxes within your business model can yield remarkable advantages, particularly in the field of artificial intelligence and machine learning. Here are some steps to facilitate this integration:
1. Identify Your Business Needs
Understand the requirements of your projects and how bounding boxes can enhance your machine learning models. Whether it is for automating processes, improving product visibility, or enhancing customer interactions, aligning the use of bounding boxes with business objectives is vital.
2. Train Your Team
Invest in training programs for your annotators to familiarize them with effective bounding box creation techniques and annotation tools provided by platforms like KeyLabs.
3. Implement Iterative Feedback Mechanisms
Establish feedback loops where team members can share insights and experiences regarding the effectiveness of bounding boxes, promoting continuous improvement in the annotation process.
Conclusion
The use of bounding boxes in data annotation is transformative for businesses aiming to leverage artificial intelligence. By understanding their critical role and applying best practices, businesses can improve the accuracy of their machine learning models significantly. With platforms like KeyLabs, organizations can streamline their annotation processes and ensure high-quality datasets that transform raw data into actionable insights.
Investing in the right data annotation tool is not just an operational choice; it is a strategic decision that could dictate the future success of your AI initiatives. Embrace the power of bounding boxes today and drive your business towards enhanced efficiency and innovation.